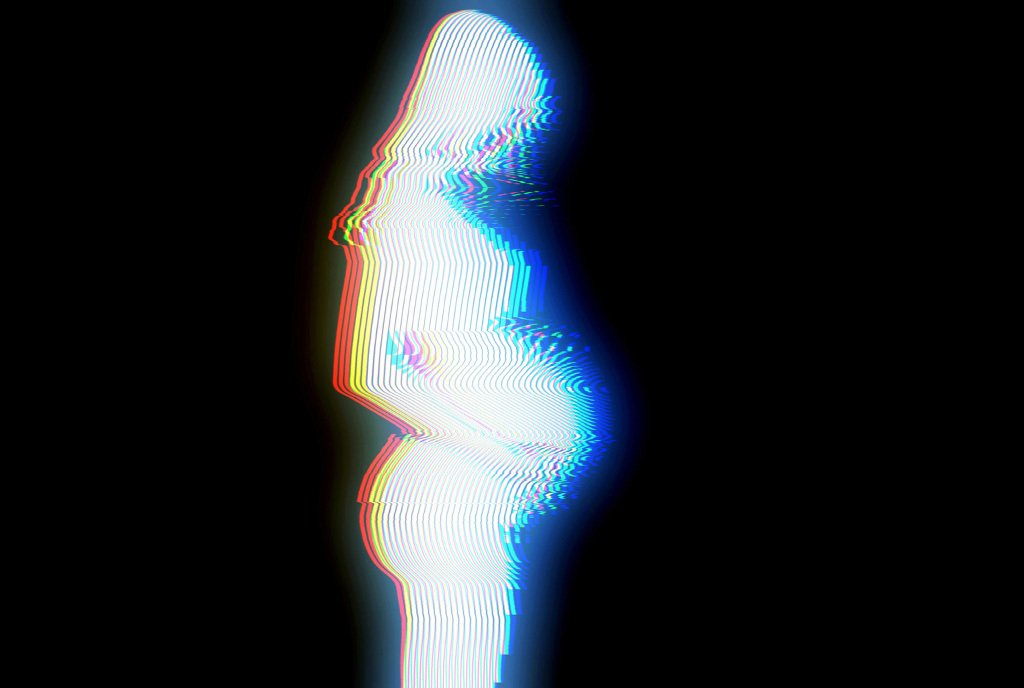
A new era of artificial intelligence (AI) is upon us. Increasingly powerful machine learning technologies coupled with large quantities of data collected at an unprecedented scale and depth have already profoundly transformed entire industries. They will likely transform many more dimensions of everyday life, including how our fundamental social interactions play out in private and public.
While many of these advances promise significant social and personal benefits, there are serious concerns about the emerging harms of AI technology, including risks of amplified inequality at the intersection of class, race, and gender. What roles can organizational investors and the entire nonprofit ecosystem play in equitably shaping AI’s socioeconomic impact?
Some nonprofits like the Surveillance Technology Oversight Project and the Center for Democracy and Technology already address algorithmic inequality through regulatory advocacy, public education, cultural interventions, and research. Yet, an overlooked but complementary approach is to leverage investment capital—in terms of organizational investors providing funding and managing their institutional investments and finances.
While many of these advances promise significant social and personal benefits, there are serious concerns about the emerging harms of AI technology, including risks of amplified inequality at the intersection of class, race, and gender.
Rhia Ventures has employed this corporate stewardship approach in the maternal and reproductive health space, engaging companies in dialogue and action about preventing and mitigating the harms of AI and commercial data practices, which impact billions of people worldwide. We have worked with multiple investors, including foundations, pension funds, investment firms, religious institutions, and individuals, to encourage large companies to take action on these issues with positive results.
Based on our successes with these strategies, we believe the nonprofit ecosystem can play a more active role in using its capital to ensure the impending social effects of AI across many spheres align with its collective missions and values.
Artificial Intelligence, Bias, and Inequality
One should first understand what fuels artificial intelligence inequality to develop appropriate investment and corporate engagement strategies. AI is dependent on a network of algorithms. These algorithms are essentially rules for how a machine operates on its own or with limited human assistance, providing instructions about how to learn from data and make decisions. Algorithms do so by automating these tasks at a robust scale and speed. With more information samples for learning, algorithms can adjust to changing conditions and improve performance.
Algorithms used in AI systems may also produce bias due to how the data are collected or analyzed. Data samples can be incomplete, contain irrelevant information, or reflect historical prejudices. The algorithms can also mirror and compound even small biases from humans who train or supervise them. For instance, a data scientist may recommend that an algorithm filter out certain types of information or make decisions based on information not representative of reality. In one example, an algorithm could be programmed (or “taught”) to conflate data about arrests in a neighborhood as the leading or only indicator of criminality in that neighborhood without factoring in historical disparities in how particular communities are policed. If these external biases creep into the data or automated systems, the algorithm will learn to replicate and amplify existing inequalities.
Even when artificial intelligence is programmed to avoid making decisions based on race, ethnicity, or gender, it can learn to bypass these restrictions by looking at proxies.
Machine repetitive learning may also create feedback loops that emphasize specific selections while excluding others. Take music streaming apps as an example. The app will learn about a user’s preferences based on selections that the user makes, such as listening to specific artists or songs. However, the algorithm powering the app might not consider that the algorithm itself selected its initial recommendations. Thus, the machine’s outputs become part of its input, and the app will suggest artists or songs similar to those initially presented by the algorithm, ultimately influencing the user’s relationship with music.
Political scientist and technologist Virginia Eubanks has revealed how these feedback loops negatively affected public services such as automated housing services for unhoused individuals in California, social welfare eligibility decisions in Indiana, and preventative child protection interventions in Pennsylvania. I have also co-written about how these AI biases can impact criminological outcomes for Black and Latino-identifying individuals, likely infringing upon their constitutionally protected liberties.
Even when artificial intelligence is programmed to avoid making decisions based on race, ethnicity, or gender, it can learn to bypass these restrictions by looking at proxies. Just a few years ago, Reuters reported how Amazon discontinued an experimental automated hiring tool after finding that the algorithm discriminated against women.
To recruit the best candidates for technical positions, Amazon developed an algorithm that would review resumes and select candidates for company management to interview. However, the algorithm was trained on data from the company’s existing pool of predominantly male software engineers. As a result, the AI hiring tool trained itself to disadvantage female applicants as it tried to maximize traits in new applicants that were most similar to traits of past successful hires.
Even after being programmed to correct for demographic characteristics, the algorithm trained itself to identify proxies for gender in applicant resumes, such as graduating from a women’s college, participating in associations frequented by women, or using words and terms that the algorithm determined were likely to be more frequently used by women. The algorithm became even more discriminatory against women than past hiring managers since the algorithm had “learned” that previous successful hires were not women, based on its independent analysis of company historical data. There are worrying reports that similar automated processes may be plaguing the hiring practices of other industries, primarily disadvantaging traditionally minoritized individuals and entire communities.
The intersectionality of race, gender, and class on these issues is especially palpable in healthcare, where AI technology is commonplace for making decisions about resource deployment, diagnosis of illnesses, and treatment. For example, a discriminatory AI could amplify existing health inequalities by recommending cardiac catheterizations more often for White men who are already more likely to have the procedure than Black women with the same symptoms.
Sign up for our free newsletters
Subscribe to NPQ's newsletters to have our top stories delivered directly to your inbox.
By signing up, you agree to our privacy policy and terms of use, and to receive messages from NPQ and our partners.
Similarly, a recent study found that an algorithm predicting the risk of vaginal birth for a patient who has previously undergone a cesarean section produced a lower score for patients who identified as Black or Latina relative to their White counterparts. These patients were thereby often denied the health benefits of a successful vaginal delivery and put at higher risk of pregnancy-related complications. There are also worries that algorithms used for pain treatment could replicate or even amplify historical biases against Black women falsely perceived as experiencing less or having a higher natural tolerance for pain.
The Data Race
The proliferation of artificial intelligence has generated a robust demand for data. This has resulted in a culture of digital surveillance for data extraction purposes, which companies justify as being done with the consent of individuals, but it is a practice better characterized as pseudo-consent, lacking transparency, ease of comprehension, valid alternatives, or the other key elements of genuinely voluntary informed consent.
Indeed, a 2019 Pew Research Center survey found that while most Americans are apprehensive about their digital privacy, the vast majority are not diligent about scrutinizing policies and terms of service they regularly encounter. “…[O]nly about one-in-five adults said they always (9%) or often (13%) read a company’s privacy policy before agreeing.” Notably, only 6 percent of adults said they understand “a great deal what companies do with the data collected,” while more than half stated that they understood “very little or nothing” about what is being done with their data.
Even with the general absence of public understanding of how and why personal data are collected, many companies have increased their tracking of consumer communications, locations, internet activity, and other unique identifiers. Some businesses use this information to generate inferential consumer data, creating detailed dossiers about their attitudes and behaviors. This sensitive information often ends up in brokered databases, sold for purposes that individual users would have never consented to in the first place.
For example, when companies are acquired or bankrupt, the data they have amassed is often sold without consumer input. These data brokerage practices are often baked into a company’s business model. Last year, the Federal Trade Commission initiated legal action against data broker Kochava upon claims that it was illegally collecting and selling the geolocation data of tens of millions of consumers, which could be used to trace their movements to and from sensitive locations such as reproductive healthcare clinics, domestic violence shelters, and places of worship.
While this data can help companies enhance and customize products and services, it can also expose individuals to severe risks. In 2022, Meta, the parent company of Facebook, provided a local Nebraska police department with private Facebook messages between a mother and daughter that served as the basis to charge both with felony crimes related to the alleged illegal termination of the daughter’s pregnancy. Based on those messages, both mother and daughter were criminally convicted and are serving prison time.
Despite an emerging appetite to tackle some of these issues, regulators in the United States have failed to catch up. No comprehensive federal law protects us against irresponsible AI, and very few laws govern data collection. The scale of available data combined with the efficiency of AI technology will only amplify inequalities that many social movement organizations are meant to protect against. Urgent nongovernmental action is needed simultaneously as the nonprofit ecosystem influences lawmakers to establish a robust regulatory framework.
Without the nonprofit ecosystem’s deliberative attention to these issues, as they emerge at this critical historical moment, other decision-makers will shape this social revolution without our input.
Leveraging Capital for Good
The nonprofit ecosystem is deeply implicated in the problems that stem from the rapid adoption and increase of AI. By using investment capital in new ways that complement existing approaches to tackle these issues, nonprofit institutions can bolster their missions and impact.
One way the nonprofit ecosystem can leverage capital is through shareholder advocacy. In many instances, endowment managers and individual stockholders can submit proposals to public companies in their portfolios to request actions by company management on AI issues. These proposals serve as an accountability mechanism whereby investors can seek public disclosures of information, adopt harm reduction frameworks, and conduct risk analyses focusing on traditionally underrepresented and disadvantaged groups. For example, a shareholder filed a proposal in 2022 seeking to assess the human and civil rights risks and harms of augmented reality products. Institutional investors submitted nine shareholder proposals for the 2023 proxy season addressing corporate data handling practices that may facilitate prosecuting individuals exercising their reproductive rights. This spring, shareholders will vote on numerous AI proposals, spanning topics such as risk oversight, executive compensation, and transparency.
Shareholder proposals can open doors for dialogue and meaningful engagement with company leadership on investment risk management, often resulting in impactful changes to corporate governance and behaviors. The proposals can be withdrawn in exchange for company actions requested by shareholders. If no withdrawal agreement is reached, the proposals go to a vote by shareholders, a majority of which would strongly signal that the company should adopt the requested course of action.
Proposals that amass strong support—even if not a majority—can also encourage companies to adopt the requested demands. For instance, shareholder proposals have proven highly effective in advancing employment protections for LGBTQ employees, despite not always achieving majority support. Overall, these shareholder proposals have the potential to raise important investment issues and positively influence the behavior of large businesses driving algorithmic inequality, especially in the absence of regulation.
Although shareholder advocacy alone cannot solve AI inequality, it can complement existing strategies and edge companies forward, enhancing the purpose of endowment money and extending the reach and influence of philanthropies. In doing so, organizational investors can ensure their values help shape the AI revolution. Without the nonprofit ecosystem’s deliberative attention to these issues, as they emerge at this critical historical moment, other decision-makers will shape this social revolution without our input.