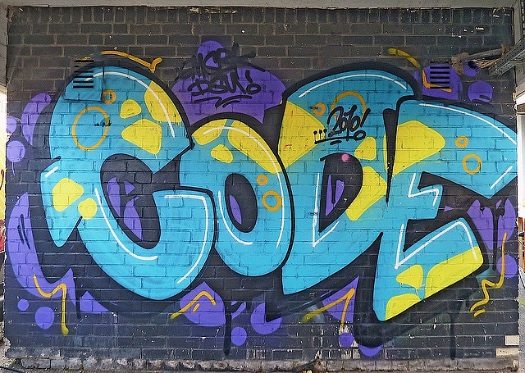
June 12, 2017; MIT Technology Review
The reality of implicit bias has been acknowledged and even used in legal proceedings to clarify the role of bias in assessing guilt or innocence. Many of us in the nonprofit sector who are committed to equity and justice understand now that many of the decisions that matter to us are often made implicitly. As we overlap this reality with the increasing use of data and predictive systems, we’re seeing that bias show up in algorithms.
When ProPublica, “a Pulitzer Prize-winning nonprofit news organization,” compared risk assessments by COMPAS, a risk assessment software being used to forecast crime, according to a recent MIT Technology Review article by Matthias Spielkamp, it found that
The algorithm “correctly predicted recidivism for black and white defendants at roughly the same rate.” But when the algorithm was wrong, it was wrong in different ways for blacks and whites…“blacks are almost twice as likely as whites to be labeled a higher risk but not actually re-offend” and whites “are much more likely than blacks to be labeled lower risk but go on to commit other crimes.”
Automated decision-making systems (ADM), as these technologies are known, are used extensively outside the justice system. Online personality tests are used to determine whether someone is good for a job. Credit-scoring algorithms are used to determine mortgages, credit cards, and even cell phone contracts. Online shopping platforms charge different prices to different customers for the same product.
To complicate matters further, even though algorithms carry our cultural biases, they also mitigate against them. Much decision-making research has demonstrated bias is at work, undetected, in most human interactions. The MIT Technology Review writes, “Human decision making is at times so incoherent that it needs oversight to bring it in line with our standards of justice. As one specifically unsettling study showed, parole boards [in Israel] were more likely to free convicts if the judges had just had a meal break…An ADM system could discover such inconsistencies and improve the process.”
Sign up for our free newsletters
Subscribe to NPQ's newsletters to have our top stories delivered directly to your inbox.
By signing up, you agree to our privacy policy and terms of use, and to receive messages from NPQ and our partners.
According to Spielkamp, it is hard to figure out how ADM systems encode bias because “the systems make choices on the basis of underlying assumptions that are not clear even to the systems’ designers.” He’s not ready to give up on algorithms. The problem is not with the algorithms, but with the programmers. In fact, he thinks algorithms can increase fairness if programmed to do so. He proposes that “lawmakers, the courts, an informed public—should decide what we want such algorithms to prioritize…Democratic societies need more oversight over such systems than they have now…What’s important is that societies, and not only algorithm makers, make the value judgments that go into ADMs.”
For example, COMPAS uses a questionnaire about a defendant’s “criminal history and attitudes about crime” to determine “risk scores.” Spielkamp, who is also a co-founder of a Berlin-based nonprofit advocacy organization called AlgorithmWatch, which helps people understand the effect the impact of ADMs asks, “Does this produce biased results? […] Are we primarily interested in taking as few chances as possible that someone will skip bail or reoffend? What trade-offs should we make to ensure justice and lower the massive social costs of imprisonment?” Further, “if we accept that algorithms might make life fairer if they are well designed, how can we know whether they are so designed?”
MIT graduate student Joy Buolamwini’s TEDxBeacon Street Talk, “How I’m fighting bias in algorithms,” highlights the key bias points and identifies solutions. As a Black woman studying facial recognition, she quickly learned that computers often don’t recognize black faces because they are programmed to learn by programmers who are not diverse, so the machine learns and adapts to white features and, one could assume, experiences. She was surprised to encounter the same problem when she was in Hong Kong. She learned they had used the same generic facial recognition software used in the U.S. and realized that bias travels exponentially this way.
She calls algorithm bias “the coded gaze.” She said, “Algorithmic bias can…lead to exclusionary experiences and discriminatory practices.”
The solutions she proposes are not that difficult. She calls it inclusive coding and lays out the principles of the Incoding Movement.
- Who codes matters—Create inclusive code by employing inclusive coding strategies using full spectrum training sets. In other words, have equal representation of the different racial and ethnic groups.
- How we code matters—Are we factoring in fairness as we’re developing systems?
- Why we code matters—We have the opportunity to unlock greater equality if we make social change a priority and not an afterthought.
Spielkamp asserts that democratic societies need to now determine the transparency we expect from automated decision systems and who will be held accountable. Nonprofits, as organizations set up for the public good, play a critical role in public decision-making. Also, local governments are seeking to become more data-driven at the same time that there is a demand for racial equity, and those overlap nicely. This decision-making point about public decision-making is very important. It will contribute to the world we create together.—Cyndi Suarez