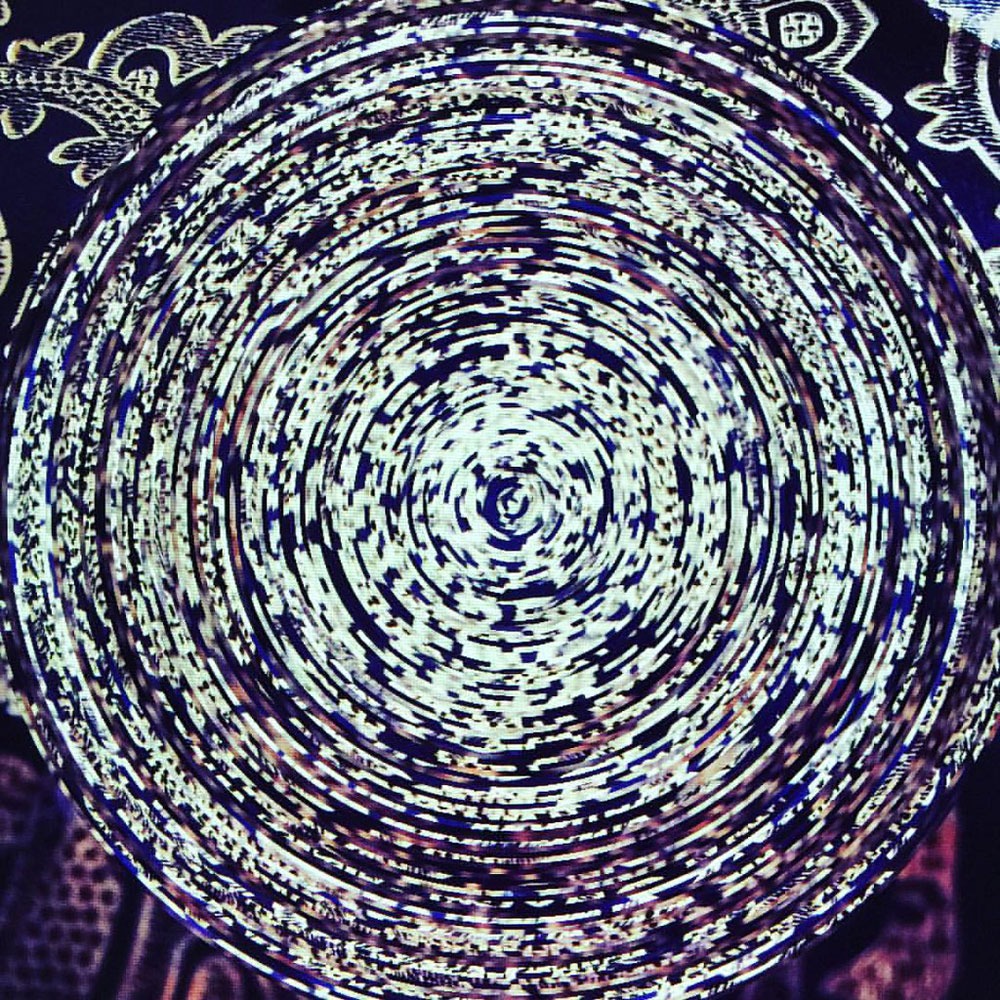
July 1, 2019; Marshall Project
As Beth Schwartzapfel writes for The Marshall Project, whether a person is released after arrest increasingly depends on risk assessment tools that are better known as “algorithms.” Indeed, Schwartzapfel reports that “more than a quarter of Americans live in a jurisdiction that uses a risk assessment pre-trial, up from just 10 percent less than a decade ago, according to the Pretrial Justice Institute, which encourages use of the measures.”
These tools, notes Schwartzapfel, “plumb your history, demographics, and other details to spit out a score quantifying how likely you are to commit another crime or to show up at your next hearing.” As NPQ has observed, these algorithms often embroider racial bias into the weave of seemingly scientific clothes.
A new study, titled Beyond the Algorithm: Pretrial Reform, Risk Assessment, and Racial Fairness, confirms this. The study is funded by Arnold Ventures and written by Sarah Picard, Matt Watkins, Michael Rempel, and Ashmini G. Kerodal of the New York City nonprofit Center for Court Innovation. As Watkins, one of the coauthors, observes, one key finding from the report is, “There’s no way to square the circle there, taking the bias out of the system by using data generated by a system shot through with racial bias.”
As the study also shows, however, there are ways to use algorithms, combined with different charging norms, to achieve dramatically more equitable results. Schwartzapfel notes that the Center’s study builds on research published three years ago from ProPublica about Broward County in south Florida. ProPublica found that algorithms in Broward County led to a system where Black defendants were twice as likely as white defendants to come up as “false positives,” labeled “high risk” yet not committing another crime. Meanwhile, Schwartzapfel adds, “White defendants who went on to commit another crime, by contrast, were more likely than blacks to be labeled ‘low risk.’”
The Center for Court Innovation study, Schwartzapfel explains, “used nine questions focused on the current charge and past interactions with the justice system, and applied them to all Black, [Latinx], and white people arrested in New York City in 2015—more than 175,000 people. They then followed up two years later to see whether the tool’s predictions were accurate.”
Unlike in Broward, the Center’s nine questions were theoretical—but the bias found was similar, as Schwartzapfel explains:
Among those who were not re-arrested, almost a quarter of black defendants were classified as high-risk—which would have likely meant awaiting trial in jail—compared with 17 percent of Hispanic defendants, and just 10 percent of white defendants.
Sign up for our free newsletters
Subscribe to NPQ's newsletters to have our top stories delivered directly to your inbox.
By signing up, you agree to our privacy policy and terms of use, and to receive messages from NPQ and our partners.
Schwartzapfel notes that this outcome occurs because racially disparate policing over decades in Black neighborhoods leads to more “false positives” in the form of “arrests of people who turn out to be innocent of any crime—as well as convictions that wouldn’t have occurred in white neighborhoods. And because risk assessments rely so heavily on prior arrests and convictions, they will inevitably flag black people as risky who are not.”
In short, “it’s racism in, racism out.” What, then, can be done?
You could throw out algorithms, but “business as usual, without the use of risk assessment, results in over-incarceration and racial bias in incarceration,” notes Julian Adler, the Center’s director of policy and research. Under the status quo, the study authors note that 31 percent of Blacks, 25 percent of Latinxs and 22 percent of whites were detained.
A second approach, the authors note, would be risk-based. Under this scenario, the study found that 22 percent of Blacks, 16 percent of Latinxs and 10 percent of whites would be detained. Fewer people are held in jail, but the racial disparity is actually wider than the status quo.
The authors’ third—and preferred—strategy would involve what they label a “hybrid charge and risk-based approach.” As Schwartzapfel explains, “In this scenario, judges would only consider jail for those charged with a violent offense or domestic violence. Anyone charged with a misdemeanor or non-violent felony would automatically go home. Judges would then use risk assessment for the more serious cases, only jailing those deemed moderate- or high-risk.”
This, the study indicates, would reduce “overall pretrial detention by 51 percent compared to business as usual and nearly eliminate disparities in detention, with Black and white defendants both detained at a rate of 13 percent, compared to 14 percent for [Latinx] defendants.”
In their report, the authors conclude, “Too often the debate over risk assessments portrays them as either a technological panacea, or as evidence of the false promise of machine learning. The reality is they are neither. Risk assessments are tools with the potential to improve pretrial decision-making and enhance fairness. To realize this potential, the onus is on practitioners to consider a deliberate and modest approach to risk assessment, vigilantly gauging the technology’s effects on both racial fairness and incarceration along the way.”—Steve Dubb