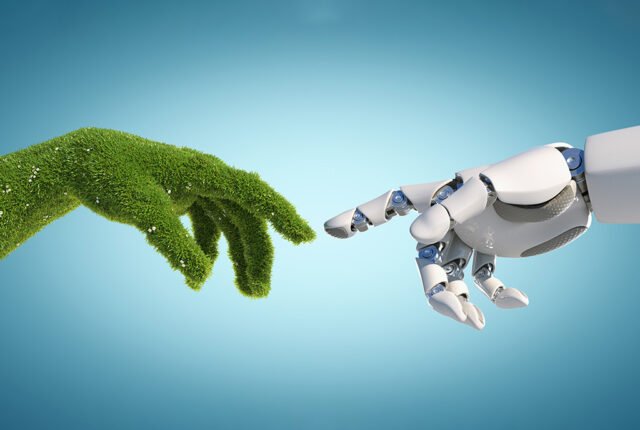
Due to its potential for market disruption, innovation, economic growth, and efficiency gains, artificial intelligence (AI) has seeped into nearly all areas of professional life. Though AI carries limitations and its misuse within health innovation ecosystems has too often entrenched inequity for marginalized communities, it also presents tremendous opportunities to transform research practices.
Before the advent of modern AI systems, environmental health researchers needed a great deal of data from natural and built environments—which could include high-volume, granular data such as satellite images generated with enough frequency to monitor change over small periods of time and data from sensors measuring environmental toxins—to understand how environments change and their relationship with human health.
These data are expensive and difficult to collect, organize, and store, and even more difficult to analyze. Accordingly, AI’s ability to efficiently collect and analyze large amounts of data has compelled many environmental health researchers to use it—even though using AI for research, ironically, carries environmental costs.
AI and Environmental Health: Signs of Progress
Though AI…has too often entrenched inequity for marginalized communities, it also presents tremendous opportunities to transform research practices.
Environmental health researchers are using AI to monitor people’s environmental exposures to toxins and severe conditions like unbearable indoor air temperatures. By integrating several large data sets into one comprehensible system, AI is also helping researchers make connections among different types of data, which can then be used to direct resources to communities most in need. A United Nations-sponsored interagency initiative, for instance, is using AI to identify underserved neighborhoods in Burundi, Chad, and Sudan.
According to the World Economic Forum, AI is also being used for other crucial climate work, including measuring changes in icebergs, which AI can do 10,000 times faster than a human can; and mapping the impact of deforestation in 30 countries.
AI supporters even argue that it is an important tool for achieving Sustainable Development Goals (SDGs). The United Nations’ SDGs include the eradication of social, economic, and environmental barriers to human health and thriving, such as eliminating poverty and hunger, providing all people with clean water and sanitation, supporting clean energy, and promoting sustainable communities.
As these systems continue to proliferate, they are “likely to need as much energy as entire nations.”
The Environmental Toll of AI
Despite existing projects that have the potential to save and improve lives, AI’s use in environmental health research is also controversial due to its own environmental costs.
Like any other emerging technology, AI has been developed and deployed in the public domain in stages. The latest of these stages is generative AI, which has had a meteoric rise in popularity with widely available and free (at least at the time of this writing) tools like OpenAI’s ChatGPT and Google’s Bard. But according to Nature, generative AI’s “environmental costs are soaring.” For instance, OpenAI’s chatbot, ChatGPT, “is already consuming the energy of 33,000 homes.” Every search uses approximately “four to five times the energy of a conventional web search.” As these systems continue to proliferate, they are “likely to need as much energy as entire nations.”
Too little attention has been paid to creating more sustainable AI systems. At the World Economic Forum, the head of OpenAI, Sam Altman, offered nuclear fusion as a solution for Generative AI’s energy use problem—though it is not the only, and arguably not the best, solution for clean energy.
Sign up for our free newsletters
Subscribe to NPQ's newsletters to have our top stories delivered directly to your inbox.
By signing up, you agree to our privacy policy and terms of use, and to receive messages from NPQ and our partners.
Energy use is not the only problem. AI systems also consume massive amounts of water. Since we refer to data storage “in the cloud,” people are sometimes not aware of the number of physical data centers needed to power advanced AI systems and the amount of water needed to keep them from overheating. Generative AI can use up to 500 milliliters of water every 10 to 50 prompts; with its wide and consistent use, this results in billions of gallons of water use each year.
AI’s capabilities to identify areas that may be affected by drought and manage water resources accordingly is undeniable, but so, too, is the way it contributes to these problems.
Due to climate change, growing populations, and megadroughts, clean freshwater is a finite resource that is becoming increasingly scarce and unevenly distributed across the globe. Approximately four billion people, or two-thirds of the current global population, are affected by severe water scarcity. Big tech is working to replenish the water it uses; however, Google, for instance, still only replaces a relatively small fraction of the water it uses to cool data centers.
Iowa and Arizona, which both host massive data centers used to fuel AI systems, are also under threat of drought and long-term water reserve shortages. As communities become more aware of AI’s water use, tensions have arisen between the companies that build advanced AI systems and the potential hosting sites where their data centers are located. Such tensions, for example, erupted in Uruguay.
Even nuclear energy—touted by Altman and others to potentially curb AI’s energy use—requires tons of water for cooling, making it an imperfect solution at best.
Somewhat ironically, several advanced AI systems are used for drought prediction and water management. AI’s capabilities to identify areas that may be affected by drought and manage water resources accordingly is undeniable, but so, too, is the way it contributes to these problems.
The Path Ahead
If we want to be kinder to our planet and protect the people most at risk from the damage that has already devastated environments all over the world, we must continue to build the political power necessary to advocate for positive change and hold multinational corporations and other responsible organizations accountable for AI’s energy and water use.
Research organizations and academic institutions should more carefully weigh the environmental costs of using advanced technology against its potential value. In human subjects research, on an individual level, researchers are asked to weigh the costs, such as potential harms and risks to research participants, against the benefits of conducting research. The contradictions associated with using AI to advance environmental health research demonstrates a need to also consider harm at the community and regional levels.
Additionally, funding agencies—nongovernmental organizations like the United Nations and the World Health Organization, government agencies, and foundations—must work to understand the environmental costs of their work and mitigate adverse impacts accordingly. As AI continues to accelerate in popularity, this may mean that funding organizations limit the number of AI projects conducted at any one time or investigate alternative methods for achieving the same objectives.
Everyone involved in research and development processes should be diligent in ensuring that as they seek to address the climate crisis, they do not, inadvertently, add to it.