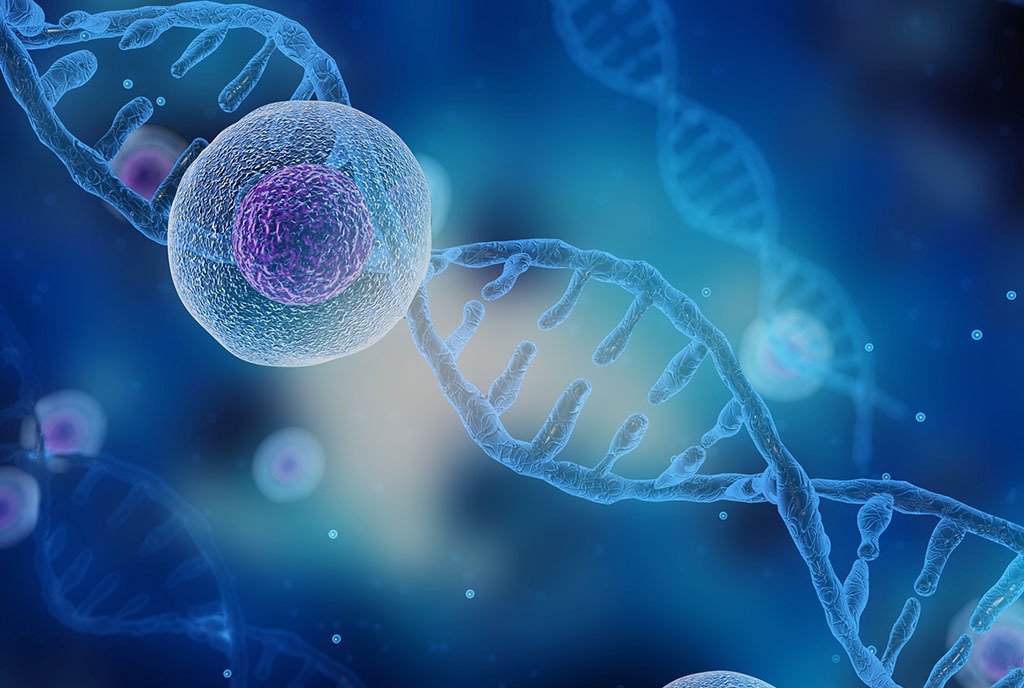
Precision medicine, which relies on genomics to understand how a person’s genetic makeup affects their health, looms large over the United States’ overburdened and underperforming healthcare system. The ability to deepen our understanding of disease susceptibility, diagnose diseases with greater accuracy, and develop tailored treatments that promote wellbeing and prolong people’s lives presents an opportunity to rectify longstanding healthcare inefficiencies and disparities. However, due to disparities in genomic data, the advent of genetically informed, personalized, or “precise” medicine may perpetuate—rather than alleviate—complex inequalities in care.
The Human Genome Project repositories consist almost exclusively of European/White genomic data.
Precision medicine’s contributions to modern medical practice are undeniable. It has led to improved treatments and therapies for rare diseases, which affect 30 million people in the United States and more than 300 to 400 million worldwide. Advances in DNA sequencing technology are leading to a new understanding of cancer and new ways of diagnosing and treating it that are saving lives. Precision medicine has also revolutionized prenatal genetic testing by making use of the small amounts of fetal DNA present in the mother’s blood, which helps expectant mothers avoid the pain and risks associated with invasive amniocentesis.
While precision medicine has opened immense possibilities for healthcare, it is stymied by the fact that the tremendous amount of genomic data collected over the last two decades lacks representation from most of the world’s population.
The Genomic Data Divide
Today’s precision medicine is largely reliant on data from the Human Genome Project (HGP), which began in 2003. The HGP has undoubtedly contributed to scientific knowledge and medical breakthroughs by laying the foundation for understanding the genomic components of disease; however, the HGP repositories consist almost exclusively of European/White genomic data.
Precision medicine utilizes whole-genome sequencing because of its ability to translate all of the 3 billion DNA base pairs that make up an entire human genome into a file made up of letters. Doctors and researchers then use tools to scan and analyze these letters for mutations, or “typos,” in the genes. The granularity of the data, coupled with AI’s ability to map millions, even billions of data points, enables scientists to identify patterns associated with certain conditions and diseases.
It [is] unlikely that underrepresented groups can benefit from the targeted care precision medicine aims to provide.
According to the GWAS Diversity Monitor (GWAS stands for genome-wide association studies), nearly 95 percent of the data in genomic studies come from European/White genomes, with a little over 3 percent coming from Asians, and less than 1 percent coming from African Americans or Afro-Caribbeans, Africans, the Latinx community, or people included in the “Mixed/Other” category. While race is a social construct that serves as a poor predictor of genetic variation, racial parity in genomic data is important because race serves as a proxy for population descriptors and other, more appropriate genetic ancestry labels.
The asymmetry in the data of whole-genome sequencing reflects imbalances in the participant pool for genomic research studies. Internationally, most genomic data comes from the United Kingdom, with more than 52 million participants; Canada, with 11 million participants; and Finland, with 5.9 million. China and Japan have approximately 2.1 million and two million, respectively. The United States has nearly 700,000, a fairly low number despite being one of the largest and most populous countries in the world.
Consequently, despite the nearly $3 billion international investment in the HGP, and subsequent investments in precision medicine initiatives, the uniformly European nature of current genomic data repositories makes it unlikely that underrepresented groups can benefit from the targeted care precision medicine aims to provide.
(Im)precise Medicine for People of Color
The gross underrepresentation of racial and ethnic minorities in the genomic data pool leads to inherently biased genomic research that inhibits our understanding of genetic variation within and across populations. This, in turn, impedes the development of tailored interventions for marginalized communities.
Diagnosing rare diseases and disorders
Disparities in whole-genome sequencing have slowed the prediction of rare diseases and genetic disorders within underrepresented populations. For example, the UK National Health Service’s 100,000 Genomes Project (100KGP) conducted a pilot study that diagnosed 25 percent of children and adults whose poor health had previously remained undiagnosed. The diagnostic yield of the pilot study, which included 4,660 participants from 2,183 families, can be viewed as an important step forward for the detection and treatment of rare diseases. However, the vast majority of the study’s participants were of European ancestry—approximately 88 percent—while South Asian ancestry accounted for 7 percent of participants, and all other racial and ethnic groups made up the remaining 5 percent.
Since the majority of data in the 100KGP comes from people of European ancestry, artificial intelligence (AI) models that use this data to identify the genetic cause of rare diseases are unable to identify causative variants specific to other ancestry groups.
Disease-causing genetic variants
Clinical genome sequencing, genetic risk scores, and targeted therapies have begun to improve the detection of genetic variants and treatments for the chronic diseases they might cause. Cardiovascular disease provides an illustrative example of precision medicine’s current limitations in identifying disease-causing genetic variants from an equity perspective.
Since genomic data is severely skewed toward European/White populations, only variants that are frequently found within these populations are linked to disease. In other words, because European/White populations are exclusively the subject of GWAS studies, the link between genes and disease is only explored within that population. However, the genes linked to a particular disease may vary among populations of different ancestry.
Sign up for our free newsletters
Subscribe to NPQ's newsletters to have our top stories delivered directly to your inbox.
By signing up, you agree to our privacy policy and terms of use, and to receive messages from NPQ and our partners.
For instance, GWAS studies have linked gene 9p21 and coronary artery disease; however, despite the hundreds of research articles published on 9p21, the association has never been replicated in populations of African or Latin American ancestry. The lack of genomic testing on racial and ethnic minorities for cardiovascular disease is even more egregious in light of the socioeconomic disparities that make Black Americans 30 percent more likely to die from heart disease than White Americans.
In other words, people whose DNA predominantly or exclusively traces back to Europe are more likely to receive targeted treatments for these conditions, though they are among the minority suffering from the disease.
Disease progression
Since racial and ethnic minority populations make up such a small portion of genetic data, the likelihood of accurate conclusions for disease progression for these groups is also low. A study published in 2022 by Oncologie, “Racial Bias Can Confuse AI for Genomic Studies,” involved applying multiple AI algorithms to a single dataset—The Cancer Genome Atlas (TCGA)—to critically examine the AI system’s ability to make accurate cancer patient survivorship predictions.
The study found that regardless of the model, AI algorithms performed reasonably well on people who are among the racial majority, but they perform poorly among racial minorities. Of the 31 cancer types included in the study, 12 were found to have a strong racial bias. In other words, AI systems, which are increasingly being deployed to make use of genomic data, are far more likely to accurately predict cancer survivorship rates and timelines—which are crucial for treatment planning, the patient’s quality of life, and resource allocation—for White patients, while underperforming for people of color.
The Reference Genome: An Incomplete Blueprint for Human Life
Much of the progress toward the diversification of whole-sequenced genomes comes from academia and government, including collaborative efforts between the two sectors. For instance, some progress has been made in diversifying the original reference genome, a standard representation of the human genome sequence that researchers use to compare DNA sequences that they generate in their studies.
In addition to creating a new, more inclusive version of the reference genome, efforts are also underway to collect genomic data from underrepresented populations.
The original reference genome largely stemmed from one person with additional data from approximately 20 more people included in the initial study. All the research participants from which the original reference genome is based were residents of Buffalo, NY, in the 1990s, where the team compiling the first reference genome ran an ad in a local newspaper asking for volunteers. The residents of Buffalo at this time were almost all European—German, Irish, Polish, and others. The reference genome, therefore, is as well.
To diversify the reference genome, researchers at the University of Washington School of Medicine are working to compile genomic data into a pangenome from 47 people whose ancestry traces back to different populations around the globe. In May of this year, the Human Pangenome Reference Consortium presented a first draft of the human pangenome reference.
Current Efforts to Collect Genomic Data from Underrepresented Populations
In addition to creating a new, more inclusive version of the reference genome, efforts are also underway to collect genomic data from underrepresented populations. In the United States, the National Institutes of Health launched the All of Us Research Program with the goal of including data from one million or more people from diverse communities.
The All of Us campaign is designed to not only include racial/ethnic groups that have been marginalized in clinical research, but also other excluded and understudied populations, including seniors, rural Americans, and people with disabilities. While genomic data from the study will not be an immediate outcome of the effort, in the future, the program will begin genotyping and whole-genome sequencing participants’ biological samples. And the data will be broadly accessible to approved researchers.
The United States serves as a stark example of the chasms between where we currently stand regarding the collection of data on whole-genome sequencing versus where we ought to be to achieve equity regarding precision medicine. Even if the All of Us campaign succeeds in its goal to conduct whole-genomic sequencing on one million people from underrepresented groups, gross inequalities in genomic data repositories will persist.
Internationally, ongoing studies are also attempting to overcome these limitations and better understand genomic variation around the world. Initiatives and targeted studies are underway in North, South and East Asia, Africa, Central and South America, and the Pacific Islands. This work is critical, as the genomic data divide also exacerbates global health inequality, positioning those descended from regions in the Global North to benefit from GWAS while leaving people descended from regions in the Global South vulnerable to misdiagnosis, poor treatments, or exclusion from precision medicine altogether.
Ensuring that underrepresented communities benefit from the advancements associated with precision medicine will require rapidly accelerating whole-genome sequencing among those currently living outside the boundaries of established clinical knowledge. By prioritizing equity, we can realize the full potential of the HGP and create a genomics revolution that benefits all individuals, regardless of their ethnic origins.